AI4Forensics Lab
A collaboration between the Netherlands Forensic Institute and the University of Amsterdam.
The AI4Forensics Lab is a collaborative initiative of the Netherlands Forensic Institute and the University of Amsterdam. It focuses on developing new AI techniques for multimodal data in a forensically sound way.
AI is expected to radically change the forensic investigation field in the coming years. More and more AI is needed to process the growing amounts of data. With the large amounts of data, we can eventually hopefully predict crimes and even prevent crimes.
More information about the AI4Forensics Lab.

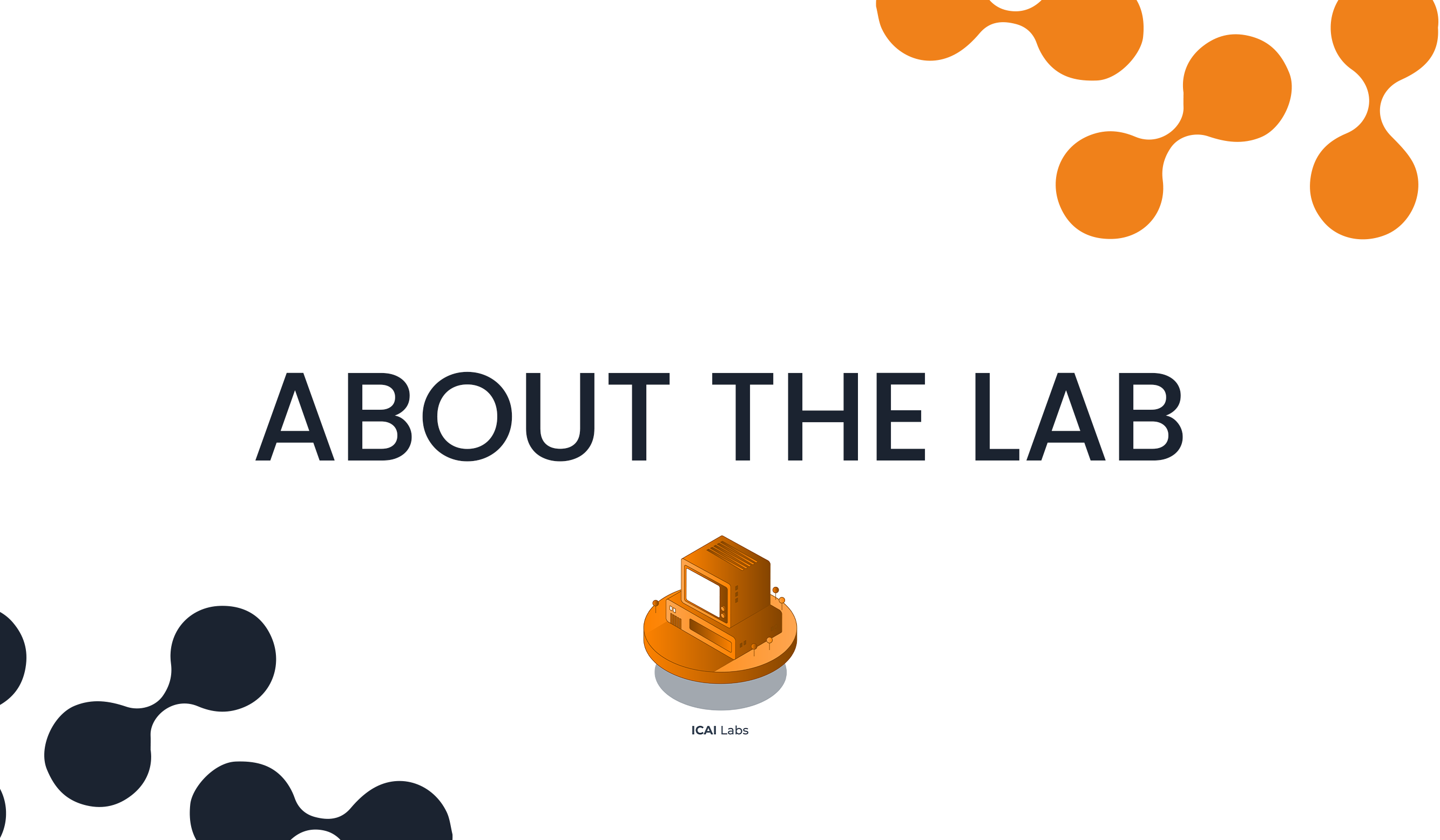
Research projects
Forensic detection of activities in heterogeneous data: Developing forensically sound methods to determine the activities a suspect was involved in by an analysis of information from for example mobile phones or wearables. These devices carry a wealth of valuable data including information on steps and distances, geolocations, speed, heartrate, messages, and images. Building on the advances in AI, in particular in multimodal and transfer learning, we aim for detection and interpretation methods which are trained on large amounts of generic data and then made specific for use in forensic practice. Innovations that we foresee are new multimodal transfer learning methods, giving such methods a probabilistic interpretation, and visualizations to make the methods and the results explainable to non-experts.
Forensic detection of deep fake videos: Develop methods for determining whether videos are real of deep fakes. We target detection techniques that stay ahead of what is possible in generating deep fakes, leveraging the best in computer vision as well as the knowledge of forensic experts who interactively can steer the analysis process through appropriate visualizations.
Forensic analysis of money streams in subversive Crime: Develop methods for the analysis of large-scale financial streams to detect and understand traces of subversive crime. Graph convolutional networks are a natural starting point to analyse such financial transactions. As traces can be individual transactions or involve many transactions we aim for multi-scale GCN methods and by using variational methods they will be given a probabilistic interpretation. Interactive graph visualizations which allow to zoom through the different scales will make the methods explainable.
Forensic analysis of signals for automatic speaker comparison: Develop methods for automatic speaker comparison in legally intercepted communications (emails, documents, telephone/video calls) to identify who are the people taking part in this conversation and what is the topic of their conversation? We aim to infer the "author" of a piece (audio or text) based on the content of speech (word usage, grammar usage, etc.) but also by their way of speaking (intonation, speed, etc.) and their use of words.
Forensic detection of steganography in images: Develop methods for detecting the presence of steganography in images and after detecting its presence gather as much information as possible about the message to get as close as possible to revealing its hidden content. Focus in on the recent deep learning based approaches for which detection methods are almost non-existent.
People
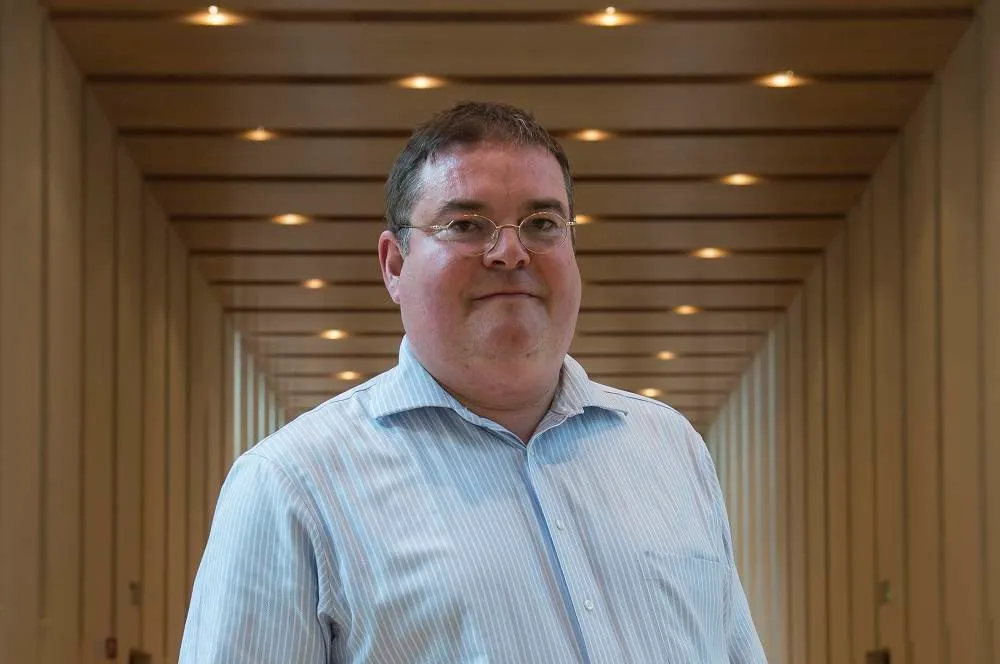
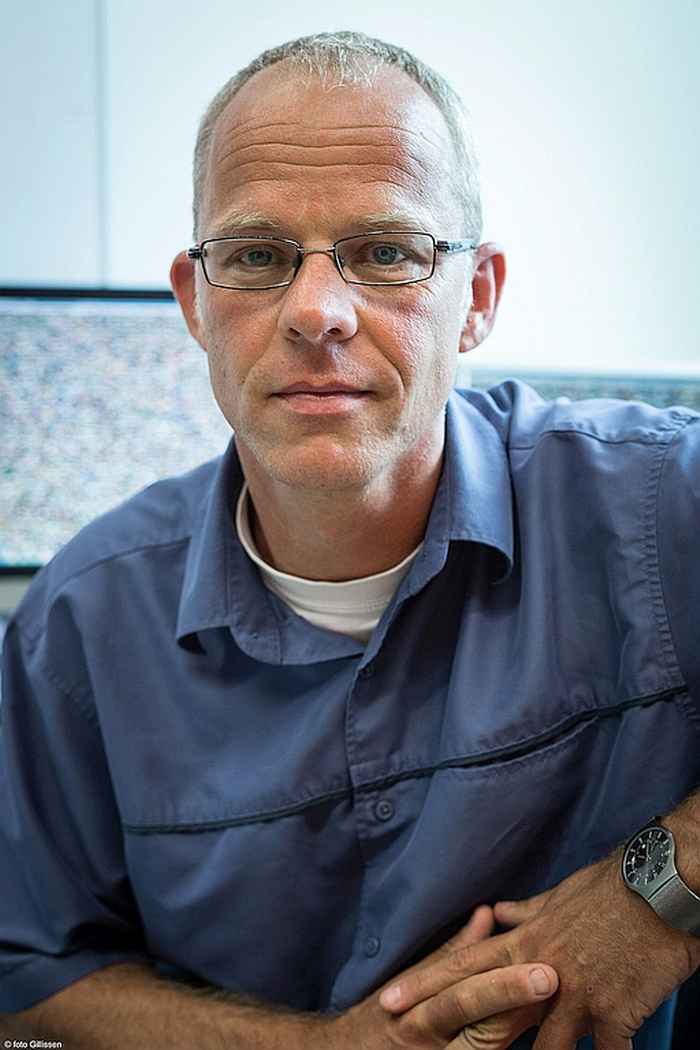
PHD Students
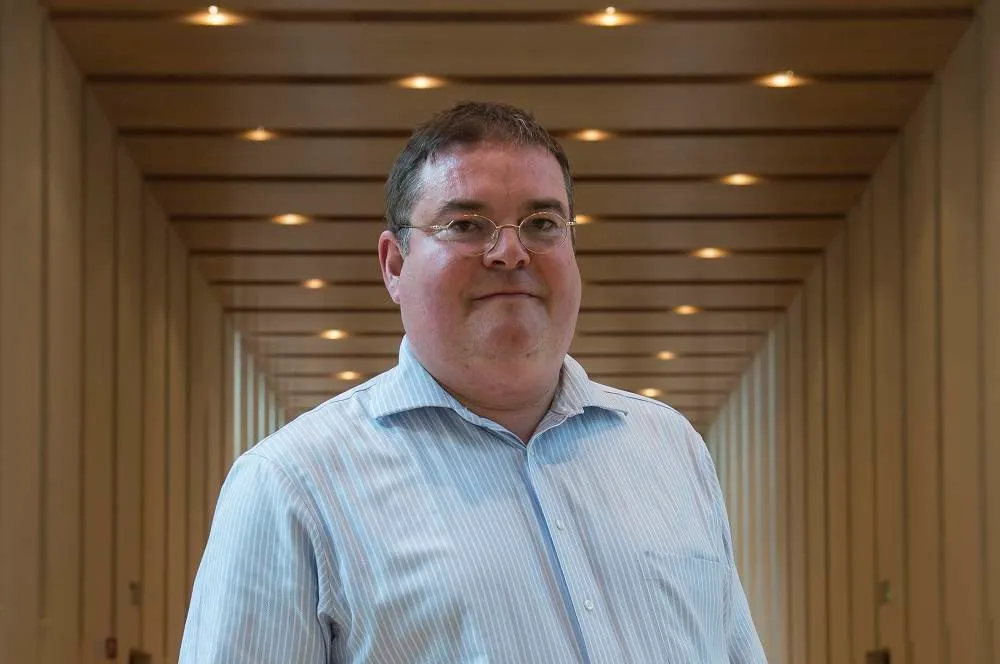
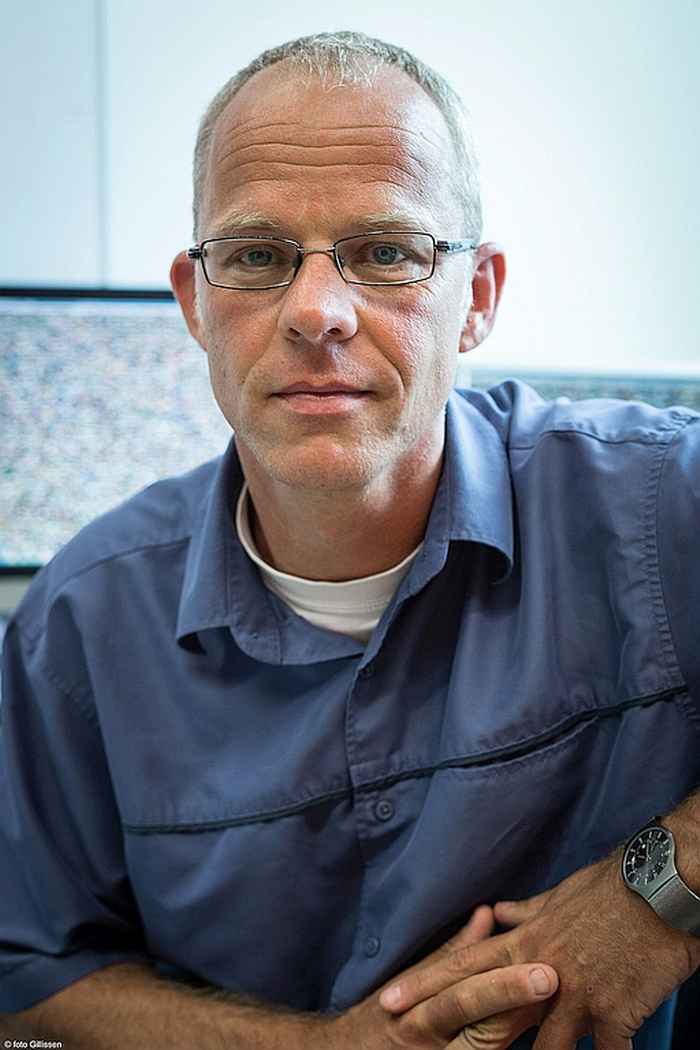
Partners
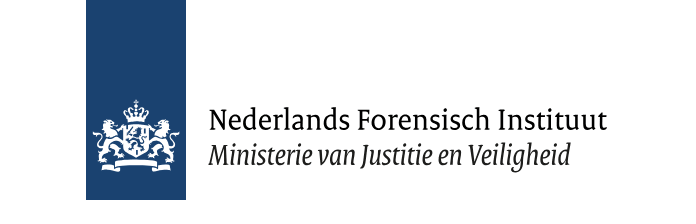
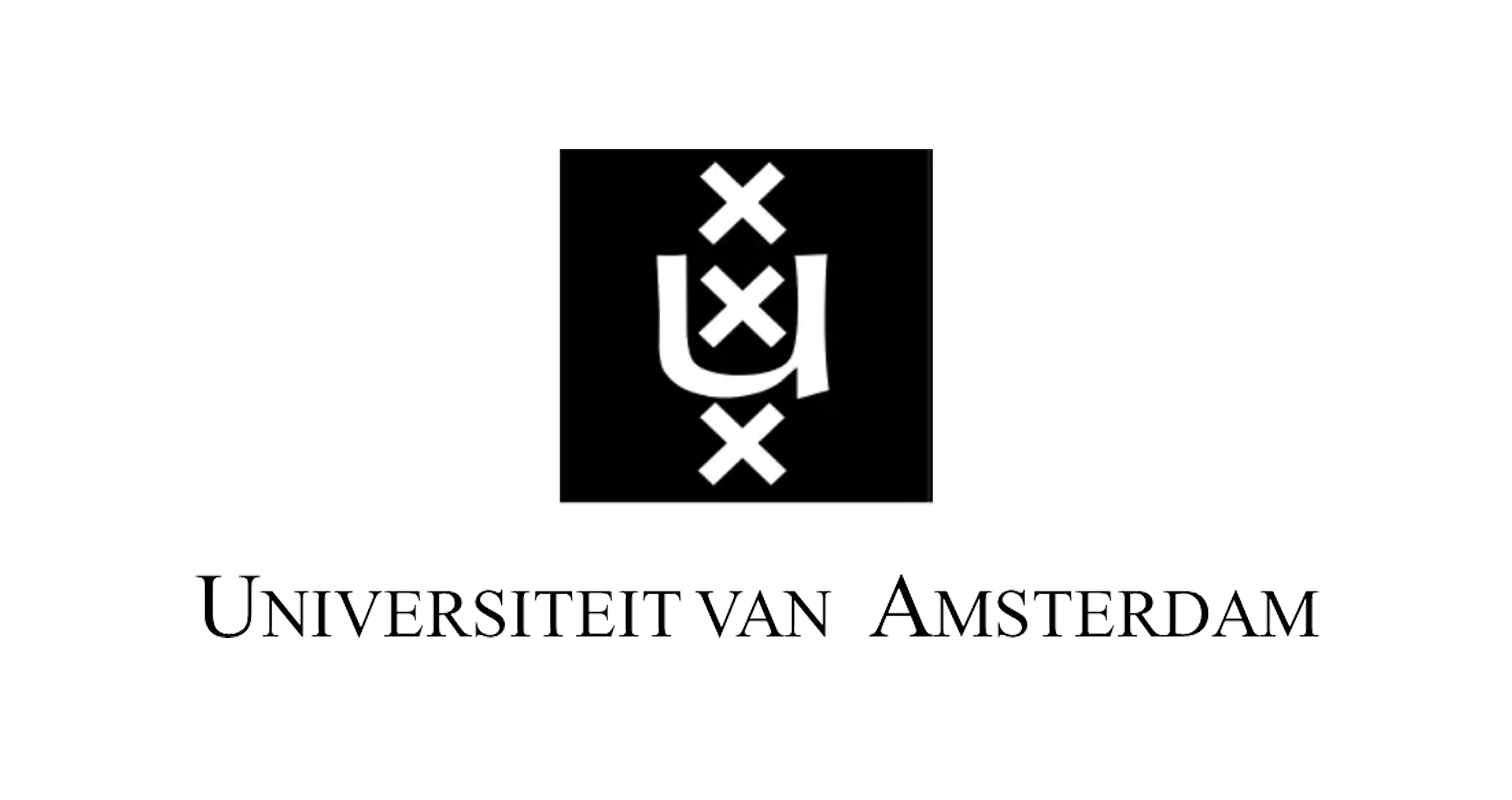